RESEARCH
Patent/ Publications
-
Saad A, Thakre S, A control unit for generating a synthetic data for an industrial machine, patent filed, (2024)
​
-
Thakre S, Karan V, Kanjarla A K, Quantification of similarity and physical awareness of the generated microstructures via generative models, Computational Materials Science , 221, 112074, (2023)
​​
-
Thakre S, Kanjarla A K, Reduced-order damage assessment model for Dual-Phase steels, Integrating Materials and Manufacturing Innovation, 11 (4), 587-606, (2022)​
​
-
Raj M, Thakre S, Annabattula R K, Kanjarla A K, Estimation of local strain fields in two-phase elastic composite materials using UNet-based deep learning, Integrating Materials and Manufacturing Innovation, 10, 444-460, (2021)​
​
-
Thakre S, Harshith V, Kanjarla A K, Intrinsic dimensionality of microstructure data, Integrating Materials and Manufacturing Innovation, 10, 44-57, (2021)
​
Patent filed
Control unit for generating synthetic data for an industrial machine
Predictive maintenance, Industrial machine, Machine learning
Method to generate faulty synthetic industrial data was designed. This approach is based on traditional signal processing techniques like Fast Fourier Transform & Wavelet analysis in combination with machine learning.
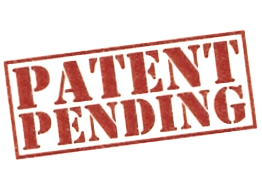
Publication online
GAN reconstructed microstructures
Generative Adversarial Networks, Similarity assessment
StyleGAN2 (Deep adversarial method) was used to reconstruct a similar microstructure dataset. The quantification of similarity was done by comparing image similarity metrics. The physical awareness was quantified by predicting the damage initiation in generated microstructures via the damage prediction model. This method helps us to explore the large microstructure space for optimal structures.
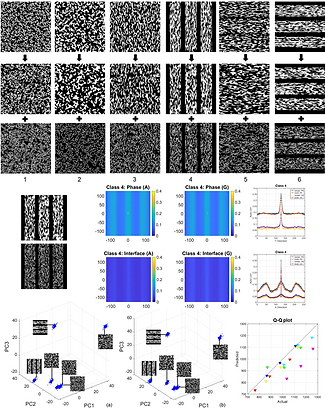
Publication online
Damage prediction in DP steels
Damage prediction, FEM, DP steels, Void growth
Ductile damage initiation in Dual-Phase (DP) steels is associated with the phase heterogeneity induced by hard martensite and soft ferrite. A microstructure and hardening sensitive reduced-order model was developed using random forest regression to predict damage initiation in DP steels. The banded DP steels showed superior damage resistance and it is further influenced by ferrite hardening.

Publication online
UNet arcitecture, Composite, Sturcture property correlations
Local micromechanical fields in the two-phase composite were predicted using UNet based deep learning architecture. The influence of microstructural features on the predictive capabilities of the model was quantified. An R squared value of 0.94 was obtained on the test dataset. The model can be used to solve the inverse microstructure design problem.
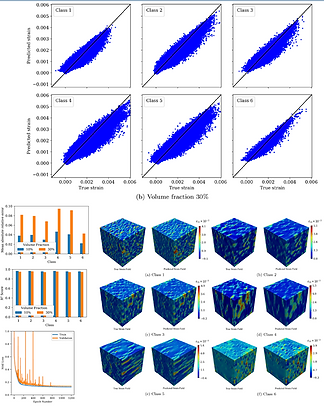
Publication online
Microstructure Informatics, Dimensionality, PCA, MDS, 2-point spatial correlations
The intrinsic dimensionality of microstructure data was estimated using a mathematical heuristic. A combination of local and global quality estimation metrics was evaluated for Principal Component Analysis (PCA) and Multidimensional Scaling (MDS). A reduced data which carried all relevant information with less computational complexity was determined.
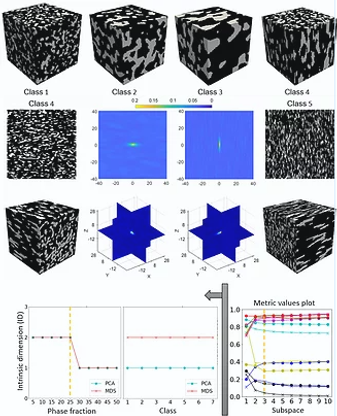
Pattern search optimization, Energy optimization, Feature engineering, Renewable energy
Windfarm layout optimization was achieved through a systemic constrained optimization by using a pattern search algorithm (MATLAB). Correlation between power and distance was used as a feature. A total of 50 wind turbine locations were optimized in order to achieve maximum power from a wind farm while considering the 'wake'. Secured bronze category (#22 out of 1529 teams).
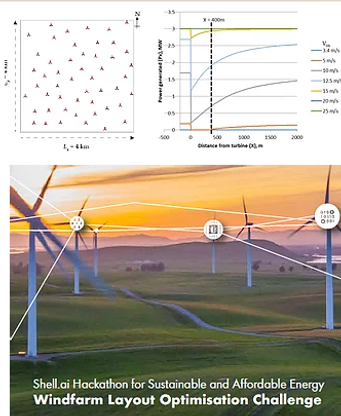